Introduction
Machine Learning (ML) is one of the most revolutionary advancements in artificial intelligence (AI), enabling computers to learn from data and make intelligent decisions without explicit programming. From personalized recommendations to self-driving cars, ML is transforming industries and redefining the way humans interact with technology. This article delves into the evolution of ML, its diverse applications, and the future trends shaping this ever-growing field.
The Evolution of Machine Learning
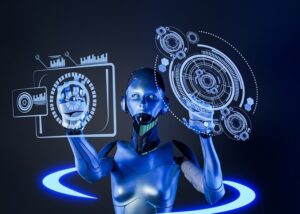
The Foundations of Machine Learning
The roots of ML date back to the mid-20th century when researchers began exploring how computers could mimic human learning. Alan Turing’s work on AI laid the foundation, while Arthur Samuel coined the term “machine learning” in the 1950s, defining it as a system’s ability to learn from experience without being explicitly programmed.
The Rise of Statistical Methods
During the 1980s and 1990s, ML evolved with the rise of statistical methods and computational power. Algorithms like decision trees, support vector machines, and neural networks started gaining popularity. These advancements enabled computers to analyze complex patterns in data, leading to significant progress in automation and predictive analytics.
The Deep Learning Revolution
The 21st century saw an explosion of ML advancements, particularly with deep learning, a subset of ML that uses artificial neural networks. With the availability of big data and powerful GPUs, deep learning has enabled remarkable achievements in natural language processing (NLP), image recognition, and autonomous systems.
Key Applications of Machine Learning
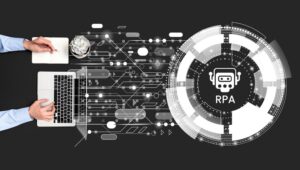
Healthcare
ML is revolutionizing healthcare by improving disease diagnosis, drug discovery, and patient care. AI-powered algorithms analyze medical images to detect diseases like cancer at an early stage. Predictive analytics helps in forecasting disease outbreaks, while chatbots assist in patient support.
Finance and Banking
Financial institutions leverage ML for fraud detection, credit scoring, and risk assessment. Automated trading algorithms analyze market trends to make data-driven investment decisions. Chatbots and virtual assistants enhance customer service experiences.
Retail and E-Commerce
ML powers personalized recommendations, customer behavior analysis, and inventory management. E-commerce giants like Amazon and Alibaba use ML to suggest products based on user preferences, increasing customer engagement and sales.
Autonomous Vehicles
Self-driving cars rely on ML algorithms to interpret sensor data, detect objects, and make real-time driving decisions. Companies like Tesla and Waymo use deep learning models to enhance vehicle autonomy and safety.
Cybersecurity
ML enhances cybersecurity by detecting and preventing cyber threats in real-time. Algorithms analyze patterns of malicious activities, helping organizations strengthen their security measures against cyberattacks and data breaches.
Education
ML-powered adaptive learning platforms personalize education by analyzing student performance and recommending customized learning materials. AI tutors and automated grading systems improve efficiency in education.
Entertainment and Media
Streaming platforms like Netflix, Spotify, and YouTube use ML to recommend content based on user preferences. AI-generated content, deepfake technology, and automated journalism are emerging applications in the media industry.
Also Read: The Transformative Power of Information Technology
The Future Trends in Machine Learning
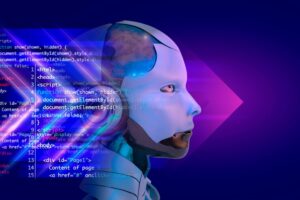
Explainable AI (XAI)
As ML models become more complex, the need for transparency and interpretability increases. Explainable AI aims to make ML decisions more understandable to humans, ensuring accountability and trust in AI-driven systems.
AI Ethics and Bias Mitigation
ML models can inherit biases from training data, leading to ethical concerns. Future advancements will focus on developing fair and unbiased AI systems that promote inclusivity and ethical decision-making.
Federated Learning
Federated learning enables ML models to train on decentralized data without compromising privacy. This approach is gaining traction in healthcare and finance, where data security is critical.
Quantum Machine Learning
The integration of quantum computing with ML has the potential to accelerate data processing and problem-solving capabilities, opening new frontiers in AI research.
Human-AI Collaboration
Future ML systems will focus on augmenting human capabilities rather than replacing jobs. AI-driven decision support systems will enhance productivity across various domains, from healthcare to creative industries.
Conclusion
Machine Learning is a transformative technology that continues to reshape industries and improve human lives. With advancements in deep learning, explainable AI, and quantum computing, ML is set to revolutionize the future of AI-driven innovations. However, addressing ethical challenges and ensuring transparency will be crucial in harnessing the full potential of ML for the benefit of society.